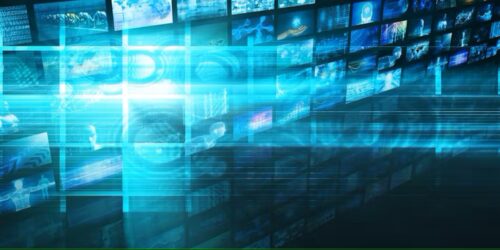
11.2.23 – SIW – Ash Young – Here are 5 video analytic options explained to better understand everything from storage to performance
Threats can come and go in the blink of an eye. A man draws a pistol in a crowded mall and then vanishes. In the security room, two, three, and four seconds pass in confusion before someone triggers the alarm, but it’s already too late.
Now, what if the security system had recognized the threat within a fraction of a second, set off the alarm in the next, and notified shop owners of its precise whereabouts before the weapon was fully drawn? In all likelihood, the outcome would not have been the same.
Security technology is changing for the better. Artificial intelligence (AI) has naturally made its appearance on the scene by doing what it does best: prescriptive analytics. Suddenly, the future of video surveillance looks very different from what it was before, and the news is just as noteworthy for those seeking optimum safety on their premises as it is for the innovators of the security industry.
There are technologies currently on the market that learn from terabytes of video footage, recognizing complex patterns over thousands of hours to process a threat—such as a lurking figure or the contours of a pistol—in milliseconds. To support such software, of course, there exists a range of different infrastructures, each of which has its own benefits, downsides, and price tags. For anyone considering an upgrade, this is an excellent time to weigh some options. Below is a brief guide to do just that.
What to Look for in Video Analytics Architecture
There are two basic components to any video analytics architecture: processing and storage.
- Processing — refers to the ability to analyze and categorize the video feed. This is the computing power of the system. The more bandwidth available, and the more readily accessible that bandwidth is, the more quickly and efficiently the AI can learn and detect threats.
- Storage — refers to how much video footage can be archived for analysis, and where that data is actually stored. Storage can take up a substantial amount of bandwidth and space if done on-premises and can be a potential liability when stored in the cloud—as discussed below.
Keep these two factors in mind throughout the list below, weighing any unique priorities against each option in order to find the optimal infrastructure.
Five Types of Analytics Architecture, from Legacy to Cutting-Edge
1. Centralized Servers
Centralized, on-premises servers, often called digital video recorders (DVRs), remain the standard surveillance infrastructure currently in use. In a DVR system, both processing and storage are handled locally. Such centralization, though, comes at a cost—namely, processing. While DVRs can efficiently manage and store footage from dozens or even hundreds of security cameras, their computing power is generally limited to doing just that. Analysis tools of any sort, not to mention AI, are severely limited as a result. Still, DVRs are time-tested, reliable, and generally more affordable than their more up-to-date counterparts.
2. Public Cloud
If bandwidth is limited—storage-wise or processing-wise—the cloud is a natural solution to consider. Public clouds allow for processing and storage to take place across dozens of servers as opposed to just one, and there’s hardly any upkeep. In theory, this is a catch-all solution and is indeed a cost-effective way to start using AI analysis. There are, however, some downsides to consider. Foremost is latency; if a system’s computing power lies elsewhere, time will always be lost communicating data back and forth. While this presents no problem for storage and AI training, a few seconds’ delay will render an AI’s split-second analysis ineffective—somewhat of a dealbreaker in the life-and-death situations at hand. Furthermore, data security risks are always present when dealing with a third-party cloud.
3. Private Cloud
A private cloud, though more costly, mitigates some of the public cloud’s weaknesses while retaining its benefits. Like the public option, private clouds allow for unmatched storage and trainability. Importantly, the owner also has full understanding and control of what happens to the data being collected and stored in this system. As with any cloud system, however, latency does remain an issue.
4. Edge Computing
Edge computing prioritizes rapid processing by placing processing power—and lots of it—as close to the camera as possible. This approach minimizes latency to the point of negligibility, allowing for real-time analysis and response by AI software in a potentially dangerous situation. All that local processing, however, comes with the cost of storage. For that reason, edge computing solutions generally need to combine forces with a public or private cloud for their storage capabilities. Since storage is the strong suit of cloud-based systems, however, this is not an unreasonable partnership.
5. Fog Computing
Finally, fog computing is the all-in-one solution of the future. As such, however, fog computing is not yet readily available, and will come at a cost when they do enter the mainstream market. The basic idea is to incorporate edge computing (that is, on-site) within a full cloud system by linking every camera directly to the cloud. While this is an exciting idea on paper, its plausibility is yet to be tested, and concerns about power consumption and data transfer remain. In any event, fog computing is an option to keep an eye on.
Conclusion
The arrival of new technologies to the security scene comes with a wide range of intriguing new options for those who want nothing but the ultimate degree of safety for their premises. With such rapid innovation, however, choosing the right solution for one’s particular needs can be an overwhelming task. To anyone who finds themself in that position, the above guide can offer the foothold needed to begin. Now, with an idea of what the video-analysis market looks like, it’s time to find the infrastructure that will ensure security to the places—and people—who matter most.
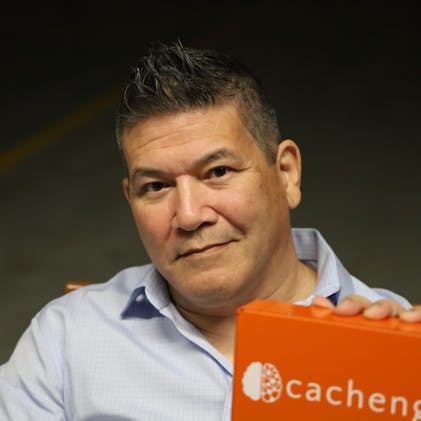
Ash Young is the CEO of Cachengo and a 30-year veteran of the data storage industry with leadership positions at multiple Silicon Valley tech companies, The company is focused on pushing analytics to the edge by providing state-of-the-art storage solutions for clients nationwide. More info at www.cachengo.com.