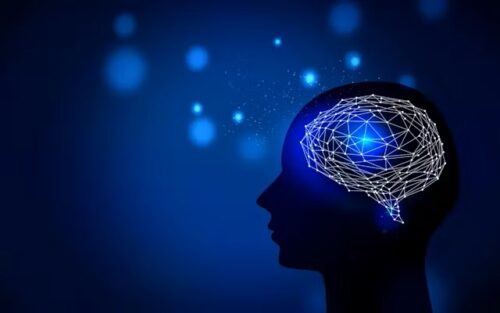
6.18.24 – SIW – Ray Bernard, PSP, CHS-III
AI needs data. Thus, the ability of any department or division in an organization (including security) to use AI effectively depends on its ability to effectively obtain and utilize data – including security.
This is the 76th article in the “Real Words or Buzzwords?” series about how real words become empty words and stifle technology progress.
Rohit Sehgal, Founder and CEO of Vincilium, recently wrote an article featured by Forbes titled, “AI Needs Data More than Data Needs AI.” I highly recommend reading the entire article, several paragraphs of which I’m quoting below with italics for emphasis.
In today’s fast-paced digital age, the buzz surrounding artificial intelligence (AI) and its transformative potential has reached a crescendo. It’s not surprising, given that AI has already made significant strides in areas like healthcare, finance and autonomous vehicles. However, as we navigate the intricate landscape of AI, it becomes increasingly apparent that AI needs data more than data needs AI.
People tend to believe that AI/ML is a magic wand that will solve all our data quality and trust issues by combing through unstructured, nonstandard and incomplete data and giving us the desired output. This is far from the truth, and I want to spend some time discussing the importance of a strong data management foundation of data quality for any AI transformation.
Data As the Foundation
and AI as the Enabler
While data plays a foundational role in AI, the reverse is not true. Data doesn’t inherently need AI to exist or be valuable. Data, in various forms, has been collected and analyzed for centuries without the need for sophisticated AI algorithms.
Data on its own can provide valuable insights and inform decision-making processes. Therefore, organizations should not blindly chase the AI hype at the cost of ignoring the importance of data management and data quality.
After I read Sehgal’s article, I began having even more thoughts about the history of physical security and IT convergence, and how the security industry was so late in understanding it and is still busy catching up.
It became even clearer to me that the security industry’s very limited understanding of information systems as a business enabler is poised to significantly undermine the utilization of AI by physical security professionals and their organizations.
Physical Security’s Use of AI
In physical security, the principal application of AI to date has been improving the accuracy of video content analysis and the reliability of alarms based on data derived from video. Other high-value uses of security video data are growing, such as in the retail sector, where video content analysis has been generating data of tremendous value for real-time enhancement of customer shopping and service experiences. Building management systems also benefit from people presence and building usage data, contributing to efficient lighting and HVAC control, as well as to preventive maintenance planning.
What generally goes unconsidered in the security domain is that the non-security uses of AI-generated data involve the automation of data flows and business processes, as well as the improvement of the focus and quality of related human activities. This could be happening within the security function itself, except that security personnel don’t have a sufficient understanding of the role that data and automation can play in security.
Outside of security, AI is very involved in workflow automation, something that physical security operations could benefit from but haven’t so far due to a lack of insight into the data aspects of security operations.
In discussions over the past year, I’ve been saying that people in the security industry, as well as the security professionals who are their end-user customers, have a low level of “data literacy.” Today I realized that I’d better define that term, or my comments won’t be of much help to anyone.
Organizational Data Literacy
Organizational data literacy refers to the capability of an organizational unit — be it an office, group or team, department, or division — to fully comprehend and effectively manage the entire data landscape relevant to its business vision, planning, and operations. This includes:
- Data Landscape Awareness
Comprehensive View: Having a thorough understanding of all data involved in and relevant to the organization’s business vision, planning, and operations, which is typically an evolving view.
Data Context: Recognizing the origin, nature, and significance of different data sets within the organizational context.
- Data Ecosystem Management
Data Flows: Understanding the various sets of data that move in and out of the organizational unit.
Interdependencies: Acknowledging how the unit’s data depend on and affect other organizational units, both internally and externally.
- Data Needs Assessment
Fulfilled Data Needs: Identifying and managing data needs that are currently being met.
Partially Fulfilled Data Needs: Recognizing data needs that are only partially met and understanding the gaps.
Unfulfilled Data Needs: Detecting data needs that are not being met and strategizing on how to fulfill them.
- Data Governance and Stewardship
Policies and Procedures: Understanding and implementing policies for data management, security, and privacy.
The ‘CIA’ Triad: Ensuring the “Confidentiality, Integrity and Availability” of data, as well as the control functions of security’s cyber-physical systems, to protect data and control functions from unauthorized access, maintain data accuracy and control effectiveness, and ensure that data and system/device control functions are accessible and operable when needed.
Data stewardship: Utilizing one or more Data Steward roles to oversee the entire data lifecycle—creating, preparing, using, storing, archiving, and deleting data—in accordance with established data governance principles to ensure data quality, integrity, and usefulness. The Data Steward role includes knowing what data the organization (or a specific portion of it) possesses, understanding where it is located, ensuring it is accessible, usable, safe, and trusted, and also advocating for data literacy.
Compliance: Ensuring that data practices comply with relevant laws and regulations.
- Data Quality Management
Data Hygiene: Maintaining high standards of data quality through ongoing practices such as data cleaning, validation, and monitoring.
Data Accuracy: Ensuring the accuracy, reliability, and timeliness of data.
- Data Analysis and Interpretation
Analytical Skills: Having the ability to analyze data to extract meaningful insights and trends.
Critical Thinking: Applying critical thinking to interpret data correctly and avoid misinterpretations.
- Data Communication
Visualization Skills: Being able to effectively visualize data to communicate insights clearly.
Storytelling with Data: Using data to tell a compelling story that drives decision-making.
- Technological Proficiency
Tool Proficiency: Being proficient in using various data tools and technologies (e.g., databases, data visualization tools, statistical software).
Data Integration: Understanding how to integrate data from different sources to provide a holistic view.
- Ethical Use of Data
Ethical Considerations: Being aware of and addressing ethical issues related to data collection, analysis, and use.
Bias and Fairness: Recognizing and mitigating biases in data and ensuring fairness in data-driven decisions.
- Continuous Learning and Adaptation
Staying Current: Keeping up to date with the latest trends, tools, and best practices in data management and analytics.
Adaptability: Being able to adapt to new data challenges and evolving technologies.
Understanding an Ecosystem
An ecosystem is a complex network or interconnected system consisting of interacting components, whether living organisms in a biological context or technological elements in a digital context, that function together to sustain and support the overall environment or system.
It is important to understand that for most organizations, the physical security function already has a data ecosystem as described in data literacy point #2 above, but it is only partially documented and hasn’t been given the consideration needed. Which means that security’s adoption of AI won’t have the foundation it needs to obtain the tremendous advantages that emerging AI capabilities can bring to physical security.
Organizational Support
Within an organization, Data Literacy typically has many cross-functional aspects, and that applies to physical security Data Literacy as well. The larger the organization, the bigger this cross-functional element is.
Many HR and IT departments have data analysts and data scientists, plus there are also people who have a good understanding of the data landscape in their functional area. New entrants to the workforce have been formally educate taught many or most of the data literacy aspects listed above.
Additionally, there is a wealth of such data available online for anyone who wishes to expand their knowledge and capabilities. The point is that physical security professionals will not be alone in tackling data literacy. They should also consider that they have an obligation to the organization to live up to the data-related responsibilities and opportunities in their own functional areas, as well as other areas to which physical security system data can be useful.
Improving Your Security Data Literacy
You can start off by sharing this article with business systems folks IT and those who head up your organization’s digital transformation initiatives, which are probably the most “data literate” business units. Learn about their visions and perspectives relating to data and its uses, including how they plan to benefit from AI and what roles data will play in that.
As I write follow-up articles about specific security industry products and services relating to this topic, I’ll update this article with references to them.
Ray Bernard, PSP CHS-III, is the principal consultant for Ray Bernard Consulting Services (RBCS), a firm that provides security consulting services for public and private facilities (www.go-rbcs.com). In 2018 IFSEC Global listed Ray as #12 in the world’s top 30 Security Thought Leaders. He is the author of the Elsevier book Security Technology Convergence Insights available on Amazon. Ray has recently release an insightful downloadable eBook titled, Future-Ready Network Design for Physical Security Systems.
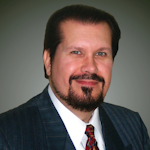
About the Author
Ray Bernard, PSP, CHS-III
Ray Bernard, PSP CHS-III, is the principal consultant for Ray Bernard Consulting Services (www.go-rbcs.com), a firm that provides security consulting services for public and private facilities. He has been a frequent contributor to Security Business, SecurityInfoWatch and STE magazine for decades. He is the author of the Elsevier book Security Technology Convergence Insights, available on Amazon. Mr. Bernard is an active member of the ASIS member councils for Physical Security and IT Security, and is a member of the Subject Matter Expert Faculty of the Security Executive Council (www.SecurityExecutiveCouncil.com).
Follow him on LinkedIn: www.linkedin.com/in/raybernard.
Follow him on Twitter: @RayBernardRBCS.