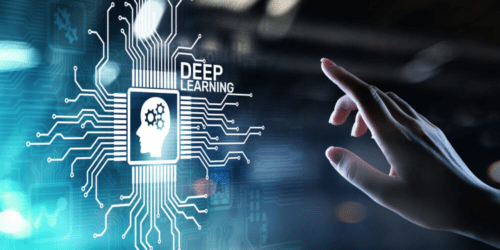
8.7.23 – Morgan Hertel
There hasn’t been a lot of focused work to leverage the use of AI to modify real-time workflow based on information from disparate systems.
When I first started in the alarm industry, the company I worked for had a small monitoring center. You could almost touch all sides of the room with both hands when sitting in the middle of the room.
It was manned with two people who were so close, they could hear each other breathing.
Back then, we had one protocol for all the intrusion alarms and all the information we fit on a 5×7 paper index card that was used to keep track of the various phone numbers and all the zones. Each card was filled out with a #2 pencil so you could erase it when a number or zone changed. Everything you needed was either in your head or on that card.
The protocol for burglary was simple: when you received an alarm, you called the police, then serially went through the call list to notify of an alarm. There were no cell phones, of course, but we had a few pagers, though they only emitted beep tones back then.
It was all pretty simple and easy. Now, fast forward 40-plus years.
While we now do a better job calling and/or sending SMS, for the most part, accounts are still worked in the same serial fashion with little room for deviation. However, we are on the cusp of all this changing.
There’s been a lot of buzz surrounding the use of artificial intelligence (AI) and Deep Learning, and, while there has been a lot of good work done for audio and video to help screen out unnecessary alarms, there has not been a lot of focused work to leverage the use of AI to modify real-time workflow based on information received from the disparate systems that exist today.
What has been done is something more prescriptive and is described variously; Rapid Response calls it Dynamic Workflow.
The idea is to be able to compile various types of measurable data elements like distance, temperature, pressure, weather, time, presence, just to name a few of the hundreds of different types of data elements, and then use these data elements to make dynamic changes to what was, for the last 100 years, a very static serial process.
How Deep Learning Could Change the Alarm Industry
There are hundreds of possible applications of this, but, to illustrate, there is the age-old situation where a patient has fallen out of a bed or chair, and though they are not injured in any way; they need assistance getting back up.
What if we could determine the location of the individuals on the call list at the time of the alarm? If we knew that, wouldn’t it make a lot of sense, in most cases, to first call and send SMS messages to the person whose proximity to the patient is closest?
Another example that quickly comes to mind is when presence detection is used, such that when an alarm is received, a query is submitted to one of the presence platforms, like Home Aware, to see the status of who is currently onsite and who recently left and how long ago.
With that information, the call pattern can now be changed to either call and SMS the person onsite or the person that just left.
In either of these two examples, two things occur: the time of resolution is shortened such that monitoring centers could then complete the events faster than before, and, more importantly, the subscriber experience is substantially better than before.
By having the automation systems surface the data, we can make better decisions and have much better information with which to speak with or text stakeholders than ever before.
Much of this is real now, is in production, and is making a difference in how alarm monitoring is being done, but it is only the precursor to what will actually become AI where hundreds of data points can be sampled to make better decisions.
Making better informed decisions will not only shorten the time to get someone help but will also make for much higher NPS scores, and, from a business standpoint, accounts will have lower attrition.
We have a lot to work to do, but the use of AI is the future—our future. It’s going to require a lot of us: education, creation of standards and standard operating procedures (SOPs), and, of course, significant software development.
If we want to continue to provide the best possible outcomes, this is what it’s going to take.
If you enjoyed this article and want to receive more valuable industry content like this, click here to sign up for our FREE digital newsletters!
About the Author
MORGAN HERTEL
Morgan Hertel is Vice President of Technology and Innovation for Rapid Response Monitoring.