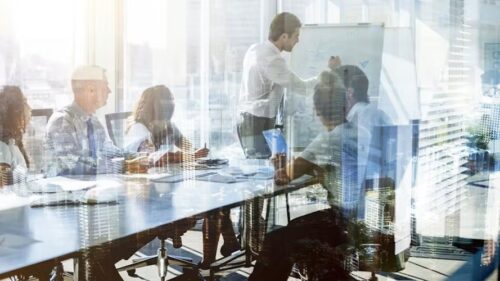
4.21.25 – SIW – Ray Bernard, PSP, CHS-III
When it comes to artificial intelligence in security systems, the integrators and consultants who can properly communicate its capabilities and underlying technology are the ones who will win the business.
The Skinny
- AI in Security Is Evolving Fast: Just like the shift from analog to digital, the rise of AI in physical security is forcing consultants and integrators to keep pace with rapidly advancing technology, particularly when interfacing with IT and data science teams.
- Security integrators and consultants don’t need to be AI experts, but they must understand and explain how AI works in their systems to gain trust and approval from increasingly AI-savvy IT and compliance teams.
- Navigating IT Reviews requires that security teams go beyond AI capabilities and address infrastructure, cybersecurity, network, cloud, and data governance concerns.
As evidenced by the many articles in Security Business just this year alone, AI development is happening at a breakneck pace – and not just for the security industry, but for the world at large. That said, these groundbreaking advancements in AI models have rapidly transformed security and video surveillance, introducing real-time situational awareness analytics that extends far beyond traditional rule-based analytics.
That has left security integrators and consultants in a quandary – and one that may feel quite familiar to those integrators and consultants who survived and remember the transition from analog to digital so many years ago. With this in mind, understanding AI capabilities and being able to properly communicate them to a customer’s IT and data analysis departments becomes critical to an integrator or consultant remaining a trusted source of technology.
If they can accomplish this feat, it will lead to increased business opportunities for both. For integrators, it will lead to expanded subscription-based as-a-service offerings, expanding their RMR. For consultants, it will position them as a trusted advisor who is continually increasing the value of an end-user’s existing investments in physical security technologies.
History Repeating Itself?
As AI capabilities keep advancing at a fast pace – faster than most integrators and security design consultants can keep up with – it brings up a troublesome aspect of the early years of physical security and IT convergence.
In the earlier days, the technical knowledge of customer and client IT personnel exceeded that of most integrators and consultants. The physical security industry developed a reputation for not knowing enough about the IT aspects of its own technology – some of which were subpar in ways that the industry’s sales and service people didn’t understand. It is critical that integrators and consultants do not allow this to happen when it comes to AI technology.
Today’s progressive security customers and clients employ data scientists and AI engineers whose AI technology knowledge far surpasses that of physical security industry folks. In much the same way that IT administrators would snicker at security software and technology sales and consulting efforts 15-20 years ago, these AI experts may very well be doing the same thing in today’s landscape.
There’s no escaping the fact that the knowledge landscape is changing so quickly that it is highly unlikely that physical security industry sales and consulting experts will be able to catch up; however, the good news is that closing that knowledge gap may not be as necessary as it was during the analog-to-digital transition.
The reason is that AI capabilities are far more proven than the clunky software efforts of ages past. Think about the degree of AI technology that would be needed to propel a self-driving vehicle – it requires hundreds of thousands of snap decisions by AI software in a minute or even a second. Now compare that to AI for video surveillance and the security use-case seems like child’s play.
This is a key aspect that didn’t exist during the digital transition. Security AI software and chip manufacturers are leaning on often open-source models that have enjoyed millions upon millions of dollars in investment and development. The security industry is simply reaping the rewards.
News stories frequently highlight AI-generated mistakes, raising concerns about its reliability; however, such risks primarily apply to general-purpose AI models and Large Language Model (LLM) generative AI used in business applications. In contrast, AI-driven security applications are narrowly focused, operating within predefined security policies and structured data environments, eliminating the possibility of hallucination or misleading responses.
It means that AI-enabled physical security applications are not prone to error, and they do not require personnel skilled in AI – just people skilled and knowledgeable in physical security. In fact, one of the key purposes of AI-enabled physical security system capabilities is to act as a significant force multiplier for existing security personnel.
While deep AI expertise isn’t necessary to use AI-enabled physical security applications, security industry professionals – from integrators to consultants to security directors – must understand how AI models function within their products. This knowledge is critical for explaining system reliability and effectiveness to AI-savvy customers and IT teams.
While security professionals don’t need to match the AI expertise of corporate AI engineers, they must be able to articulate how AI in their security solutions works, why it is reliable, and how it aligns with enterprise IT policies, including cybersecurity and data governance. This ensures IT approval for deployment within the corporate infrastructure and credibility when making security technology performance claims.
In the end, this means that history does not need to repeat itself. If integrators and consultants can simply explain the technology and what it can do, it will open the door to more business and trust in the technology to accomplish what it is designed to do.
Talk the Talk with IT Review Boards
AI-enabled physical security technologies often must pass through multiple IT review boards and/or individual reviewers within an enterprise – each with its own scope and concerns. Navigating these IT reviews requires that security teams go beyond AI capabilities and address infrastructure, cybersecurity, network, cloud, and data governance concerns.
Understanding each review board’s primary focus areas and considerations for AI-driven physical security deployments ensures a smoother and faster approval process. Here’s a closer look at the most common review boards and what they are looking for:
Architecture Review Board (ARB)
The ARB ensures new technologies align with enterprise IT architecture and long-term scalability. It evaluates system interoperability, integration with existing platforms, and infrastructure impact, and reviews data flow, performance, and redundancy requirements to maintain system stability.
The ARB’s key AI considerations: Can the AI security system integrate with existing IT infrastructure? Does the system meet enterprise architecture standards for cloud, on-premises, or hybrid deployments?
Security Review Board (SRB)
The SRB assesses compliance with cybersecurity policies, risk management frameworks, and regulatory mandates, such as GDPR or HIPAA. It evaluates encryption, authentication, access control, and vulnerability management in AI-driven security applications and ensures AI decision-making aligns with corporate security protocols to prevent unauthorized actions.
The SRB’s key AI considerations: Does the AI protect sensitive security data, such as camera footage, access logs, or biometric templates? Is the AI’s decision-making process secure, auditable, and tamper-resistant?
Network Review Board (NRB)
The NRB reviews bandwidth requirements, network load impact, and communication protocols for AI-enabled security devices. It ensures AI systems do not degrade network performance or create vulnerabilities, and assesses whether AI-driven real-time processing is best performed at the edge or in the cloud.
The NRB’s key AI considerations: Can the network handle continuous AI-driven video analytics without causing latency issues? Does AI processing occur on-site (edge computing) or in the cloud, and how does that affect data transmission?
Cloud Review Board (CRB)
The CRB evaluates AI applications hosted in public, private, or hybrid cloud environments to assess data sovereignty, cloud security, and compliance with cloud governance policies.
The CRB’s key AI considerations: Are AI security systems deployed on an approved cloud provider, such as AWS, Azure, or Google Cloud? Does AI meet cloud governance policies for storing and processing video and security logs?
Data Governance Review Board (DGRB)
The DGRB establishes policies for data storage, retention, and privacy protection in AI applications and that the data complies with corporate policies and legal requirements.
The DGRB’s key AI considerations: Does AI handle personal data responsibly, ensuring compliance with privacy laws? What is the data retention policy for AI-processed security events, and who can access them?
AI Review Board (AIRB)
The AIRB examines AI transparency, fairness, bias mitigation, and explainability. It ensures AI operates within ethical guidelines and corporate AI governance policies and assesses Agentic AI and automation levels to ensure human oversight in security decisions.
The AIRB’s key AI considerations: Does the AI security system use Explainable AI (XAI) to justify its decisions? Are AI-driven security actions – such as alarm escalation or access denial – aligned with policy and human oversight?
How to Talk the Talk
In the past, a single IT approval was often sufficient to add physical security products to an organization’s IT infrastructure. Today, AI-enabled security technologies must pass multiple IT review boards, including the Architecture Review Board (ARB), Security Review Board (SRB), Network Review Board (NRB), Cloud Review Board (CRB), Data Governance Review Board (DGRB), and even an AI Review Board (AIRB).
To get acceptance, focusing only on AI-based capabilities isn’t enough, no matter how revolutionary they may be. AI-powered security applications rely on extensive data integration, meaning deployment must align with IT infrastructure, security policies, and compliance frameworks. This requires a decent understanding of the underlying technology.
AI Transformers
Integrators and consultants must be prepared to address enterprise IT concerns, ensuring AI-driven security solutions meet both operational and IT governance requirements. With this in mind, explaining how AI model improvements enable specific physical security system capabilities – including four-dimensional full-incident situational awareness – is paramount. This starts with AI Transformers.
The T in “ChatGPT” means that it uses a “Transformer architecture” – a deep learning model designed to process and analyze sequential data efficiently by understanding relationships between words, phrases, and concepts in context.
Transformers have revolutionized AI by allowing models to handle long-range dependencies, meaning they can retain context over extended interactions. This is particularly valuable in AI-powered security systems, where AI must process and correlate video analytics, access control logs, intrusion alerts, and other security data in real-time. Unlike conversational AI models like ChatGPT, security AI applications use specialized transformers tailored for situational awareness, anomaly detection, and policy-based decision-making – ensuring high accuracy, real-time response capabilities, and conformance to security protocols.
Jensen Huang, the founder of NVIDIA, recently said: “The fundamental characteristic of a transformer is this idea called ‘attention mechanism,’ which basically says the transformer is going to understand the meaning and the relevance of every single word with every other word.”
Transformers achieve their higher levels of comprehension through specialized attention mechanisms that determine which data points are most relevant at any given moment. The three key types of attention mechanisms that Huang mentioned play a critical role in enhancing AI-driven security situational awareness: flash attention, hierarchical attention, and wave attention.
Flash attention is a high-efficiency mechanism that reduces computational overhead, allowing AI to process vast amounts of video, sensor, and log data in real time without sacrificing accuracy. Hierarchical attention organizes information at multiple levels, enabling AI to focus on broad security patterns (e.g., unusual crowd behavior) while simultaneously tracking fine details (e.g., individual intruder actions). Wave attention is optimized for continuous and evolving data streams, ensuring AI dynamically adjusts focus as new events unfold across multiple security feeds.
Breakthrough Security Capabilities
These breakthroughs in transformer technology have made four-dimensional (3D plus Time) security situational awareness possible.
Imagine that a security officer is running or driving to a point of activity and thus can’t stop to read a phone text message or examine a set of video images. State-of-the-art AI can provide full-campus as well as area-of-interest coverage at any scale or complexity of activity. It can keep security personnel informed with text, visual, and spoken word updates. That officer can now be kept updated by an ongoing AI-generated spoken narrative that provides people, vehicle, and activity audio descriptions so that the officer can focus on the immediate travel steps and be completely up to date upon arrival at the scene. The officer can also talk with people on the scene before arriving. The AI can translate between languages in real-time if necessary.
Today’s emerging AI-driven surveillance systems integrate multiple advanced AI technologies, several of which directly contribute to physical security situational awareness:
Vision Transformers (ViTs): AI models designed for image and video analysis, enabling advanced object detection, activity recognition, and anomaly detection in security footage.
Large Language Models (LLMs): Text-processing AI trained on vast datasets, allowing security AI to interpret, summarize, and generate reports based on security alerts, incident logs, and policies.
Large Multimodal Models (LMMs): AI models that process multiple types of data (text, images, video, and audio), making them ideal for correlating security camera feeds, alarm data, and spoken alerts into a unified situational awareness framework.
Long Short-Term Memory Networks (LSTMs): AI models specialized in analyzing sequences of events over time, enabling security systems to track movement patterns, detect unusual activity durations, and identify anomalies based on deviations from normal behavioral timelines. LSTMs model event progression, helping to predict security violations before they occur.
Agentic AI: AI systems capable of autonomous decision-making and action execution based on predefined policies, reducing response time in security operations by dynamically adjusting surveillance priorities and responses.
Explainable AI (XAI): AI frameworks that provide transparent, human-understandable justifications for AI decisions, ensuring security personnel understand why alerts are triggered and how AI-generated recommendations align with security protocols.
All of these AI elements support Human in the Loop (HITL), ensuring that AI-driven security systems operate with human oversight where necessary; however, Agentic AI makes HITL essential, as AI is not just analyzing data but actively making decisions and taking actions based on security policies. This elevates the role of security personnel from constant monitoring to high-level decision-making, where AI assists rather than replaces human expertise.
At the same time, Explainable AI (XAI) makes HITL precisely effective by providing clear, human-understandable justifications for AI-generated alerts, recommendations, and actions. Instead of security personnel questioning why an AI system flagged an incident, XAI ensures they see the reasoning behind every decision, enabling faster validation and more informed responses.
By combining Agentic AI’s decision-making, XAI’s transparency, and HITL’s oversight, AI-powered security systems achieve true real-time situational awareness and act as a powerful force multiplier for security operations personnel, allowing them to process vast amounts of real-time security data, make faster, data-driven decisions, and reduce response time. The key is that they can trust AI-driven recommendations, knowing they are policy-based and explainable.
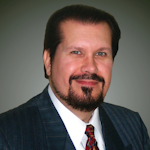
About the Author
Ray Bernard, PSP, CHS-III
Ray Bernard, PSP CHS-III, is the principal consultant for Ray Bernard Consulting Services (www.go-rbcs.com), a firm that provides security consulting services for public and private facilities. He has been a frequent contributor to Security Business, SecurityInfoWatch and STE magazine for decades. He is the author of the Elsevier book Security Technology Convergence Insights, available on Amazon. Mr. Bernard is an active member of the ASIS member councils for Physical Security and IT Security, and is a member of the Subject Matter Expert Faculty of the Security Executive Council (www.SecurityExecutiveCouncil.com).
Follow him on LinkedIn: www.linkedin.com/in/raybernard.
Follow him on Twitter: @RayBernardRBCS.