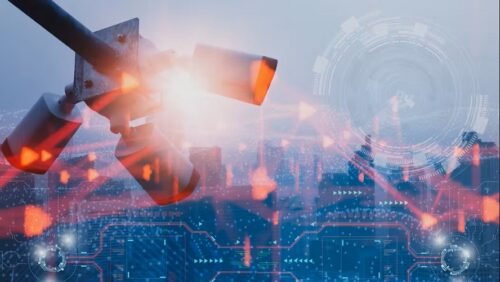
9.7.23 – SIW
Learn how artificial intelligence processing has improved, along with use-cases for integrators
Anyone who has ever installed a Ring doorbell knows the benefit of cloud-based video security. Anyone who’s used ChatGPT understands the power of artificial intelligence (AI).
Many security integrators operate where the two intersect: They implement cloud-based video solutions that use AI to analyze what they see. But as technology develops and grows more pervasive, potential inefficiencies become apparent.
Indeed, the cloud has been a revolutionary platform for a litany of applications – email, video communications, streaming media, and even generative AI chatbots. But when it comes to security applications, for which the impact is often felt in real time and far from cloud data centers, intelligence should be generated at the computing edge.
Security Applications at the Edge
Security integrators understand the edge better than most, because it is where much of their technology – IP cameras, sensors, alarms, and access controls – has always existed. Once network-enabled, such devices have become many of the “things” on the Internet of Things, positioned far from the control rooms and data centers where the data they generate is collected and analyzed.
With the advent of AI, many industries have embraced edge computing in order to locate more powerful devices in the field, closer to where data is generated and used. Industrial manufacturing uses AI alongside robotics to more quickly identify issues on the shop floor. Healthcare uses AI embedded in imaging systems to better spot life-threatening conditions and improve patient outcomes.
There are many applications of AI in security: object/people detection, facial detection, license plate detection, behavioral analysis, traffic monitoring, pose recognition, and more. Traditionally, cameras and sensors continuously capture data, sending it back to the cloud over available networks, like 5G, for processing and analysis — by people or machines. But as in other industries, processing security data closer to where it is captured is more efficient, faster, and results in better outcomes and improved privacy protection. For that reason, security solutions have begun to embrace edge AI.
How Edge AI is Different
Moving AI to the edge of security systems requires a different way of processing AI data. In data centers, AI benefits from slews of servers and CPUs for handling the intricate neural networks on which AI is based. By their nature, neural networks are non-linear. They are designed like the human brain to process information through various nodes. They have been compared to hockey teams, which pass a puck back and forth in varying patterns and intervals before shooting on goal.
In the data center, where many compute nodes are brought to bear on neural network processing, this is eminently achievable, albeit at a cost. According to Earth.org, it is estimated that ChatGPT, for example, has an annual carbon footprint of more than 8 tons of CO2 from all the server energy used for processing.
At the edge, where processors are necessarily limited in number (sometimes to just one, for instance, in a “smart” camera), traditional methods are vastly inefficient. Forcing neural networks through traditional, linear processors requires power and dissipates energy that edge devices cannot afford.
Modern edge AI processors are designed from the ground up to process neural networks the way they actually work. Rather than forcing data through a linear pipe, edge AI processors include an architecture that adapts to neural networks. In practice, such an efficient architecture is especially apt for security devices. Not only do they rapidly run AI algorithms, but they also do it with less power, resulting in emission of less heat —attributes that are important when installing an edge AI surveillance camera outdoors, for example. (since the addition of fans for cooling, for example, is not advisable in outdoor installations).
Edge AI for Security
When it comes to security applications, there are a couple of ways to deploy edge AI: It can be integrated into edge devices themselves or into an aggregation system that is located at the edge and ingests data from devices for processing and analysis.
Because video security is such a burgeoning market, there is significant interest in edge AI for vision processing. In that case, edge AI can be integrated directly into cameras or into edge boxes, which include purpose-built VMS or NVRs. Such boxes, often with multiple edge AI processors included, take video streams from standard cameras and apply AI algorithms for detection, identification and more. No need to send the video data to cloud servers for processing.
In addition to video analytics, AI can be used to improve network utilization and lower per-channel bandwidth by streaming relevant content only. AI also improves storage space by better controlling the access bandwidth to the storage elements as well as eliminating the need to store unnecessary content. Finally, AI-based analytics can also be used to spot relevant regions of interest and enable relevant triggers based on what is detected.
Video cameras with built-in edge AI have only recently been viable, in large part due to the advances in AI processing. But not only do such cameras require specialized AI processing, they also need video processing capabilities, such as image enhancement, video stabilization, white balancing, and more. Such all-in-one vision processors exist today.
And while cameras with edge AI get us as close as possible to real-time analysis and decision-making where video is captured, it is not possible – or necessary – to swap all existing cameras for AI cameras in the short term. VMS or NVR edge boxes offer a way to bring edge AI to existing security systems. Depending on application needs, they also make sense for new solutions where real-time processing is not always required.
The Role of Security Integrators in Edge AI
Security integrators play a key role in enabling edge AI. Although it helps, they do not need to understand the intricacies of neural networks and deep learning; however, their expertise in developing use-cases and needs analyses are key.
Use-cases for edge AI typically fall into two main buckets. The first involves making decisions and taking action faster, closer to where incidents happen. When an edge AI security device detects a suspicious object, for example, it can trigger alerts or initiate security measures immediately. One of the trickiest challenges in video security is re-identifying individuals as they move through different areas of a transportation terminal, for instance, or a stadium or shopping mall. In this case, an edge AI box takes feeds from cameras across different areas and rapidly detects the same person as they appear in different feeds.
The second bucket comprises solutions for intelligently processing data for later evaluation. With edge AI, such video can be processed, analyzed, and categorized before being stored, so when security teams must go back and look for a person, object or event, they can apply search terms to the stored video rather than pore over all the footage.
Ultimately, of course, use-cases are driven by need and budget. Certain critical, real-time security solutions will benefit from edge AI built directly into new video cameras and other detection devices. Others, including existing video camera deployments, will be suited to cost-effective edge boxes for aggregating and analyzing streams. Chances are most edge AI security solutions will fall in the middle, with a combination of AI devices and aggregation boxes.
Integrators, in consultation with clients, will help match this new, hybrid, edge-to-cloud AI ecosystem to each unique application. For a smart city deployment, is it enough that an edge AI system be able to detect loitering in a park at 2 a.m., or does it need to recognize the faces of the loiterers? Emerging behavioral detection technology can be powerful, but if the behavior detected is a threat to others, the edge AI solution may need to trigger an immediate – if not preemptive – response.
This article originally appeared in the September 2023 issue of Security Business magazine. When sharing, don’t forget to mention Security Business magazine on LinkedIn and @SecBusinessMag on Twitter.
Yaniv Iarovici is VP of Business Development for Hailo. Request more info about the company at www.securityinfowatch.com/53027795.