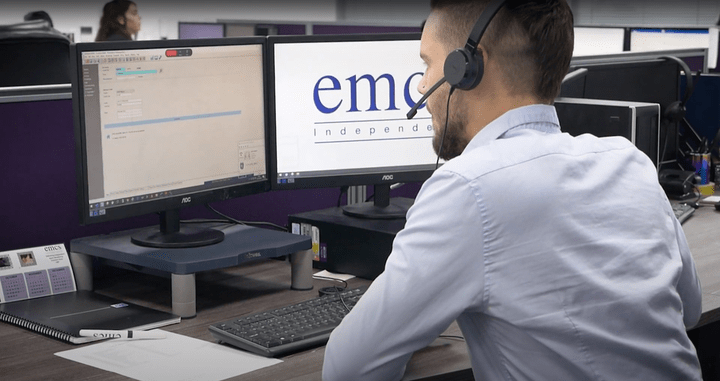
10.9.20 – SIW
This article originally appeared in the October 2020 issue of Security Business magazine. When sharing, don’t forget to mention @SecBusinessMag on Twitter and Security Business magazine on LinkedIn.
The explosive growth in the numbers and capabilities of video surveillance systems over the past 20 years has resulted in better security and protection of people and property. Unfortunately, it has also led to an explosion in false alarms, which have created often contentious relationships between businesses, central monitoring stations, first responders and city governments.
A typical central station operator is estimated to be exposed to at least three alarms per minute. With up to 95% of those alarms being false, an operator’s ability to respond to genuine activations is limited.
In addition to slower response times, false alarms are expensive. Many U.S. cities charge fees of up to $500 per incident after one or two false alarms per month to recoup some of the costs of dispatching first responders to false incidents. Continued false alarms may result in the suspension of a user’s alarm permit, leading to slow or possibly no response. Some cities bill monitoring centers and security companies for the fines, putting the providers in the position of collecting fees from their subscribers or writing them off as a loss.
AI to the Rescue
Thanks to advanced cloud-based video analytics, software-based artificial intelligence (AI), and its subsets machine learning and deep learning, technology can help filter out 90% or more of false alarms. AI software manages the repetitive tasks of identifying false alarms from thousands of cameras, enabling human operators to focus on genuine activations and more complex jobs that add value to their employer and customers.
Case in Point: As one central monitoring station grew, so did its number of false alarms; in fact, the station had reached its capacity to accept new connections – as continued expansion could impact the overall security of the existing customer base. There was a concern that genuine alarms could be delayed or missed while operators dealt with false activations.
After integrating AI-based false alarm reduction software into the central station’s operating systems and customer cameras, nuisance alarms were reduced by 90%; thus, improving monitoring operators’ performance and enabling the central station to add customers without the necessity and expense of hiring new resources.
How it Works
Most video analytics software, such as motion detection, uses what is known as rule-based algorithms. The software relies on a decision tree to test multiple hypotheses before making a prediction; however, there are too many variables in video to accommodate high-quality false alarm reduction using a relatively simple rule-based method.
Neural networks, which are inspired by the way the human brain works, provide significantly improved accuracy. The systems “learn” to do tasks by considering examples without being programmed with task-specific rules. Multiple nodes are layered together, with each analyzing various elements of an image. Once the image has passed through all nodes, a prediction is made based on the in-depth analysis.
Human engineers correct wrong answers, and over time, the neural network learns from its mistakes in a deep learning process called training. Once trained – which in this case, is determining the difference between false and genuine alarms – the network can make highly accurate predictions about new events.
It takes time and tremendous computational power to expose the neural networks to millions of images – for example, people in various poses and sizes wearing distinct clothing, all from different angles, lighting and locations. A similar process is repeated using a variety of vehicles. Training also involves thermal cameras which adds the ability to monitor events in low-light environments.
Monitoring stations that invest in AI-based technology are not purchasing a static product; rather, it is one that will continue to improve its performance over time. Field experience has shown that neural networks continue to become more accurate following deployment.
There are some key elements to look for in a false alarm reduction solution. Make sure any system is scalable, capable of efficiently handling additional cameras as a customer’s needs change. Software-based solutions are simple to remotely install from the cloud, while hardware devices typically require expensive onsite installation and are not as simple as software to upgrade. Also, ensure the solution will integrate with existing monitoring software and the a customer’s camera brands.
Data is vital to running an efficient monitoring operation. Using dashboards, managers see trends in instant software performance based on selected periods. Data usually includes the number of false vs. genuine alarms. It may also show idle cameras that need to be replaced or moved to better positions.
Other Benefits of AI and Analytics
With central stations integrating AI-based technology into monitoring operations as an extension to human verification of alarms, central monitoring stations can have confidence that they are providing their customers with an accurate and continually improving service. However, security professionals should not be looking to AI to run their monitoring operations or replace staff. Instead, it should be viewed as an extension and enhancement to augment existing services. By building a collaboration between humans and technology, security companies will provide more value to customers. In this way, AI-based software is ideal for integrator-run stations, third-party or wholesale operations and enterprise organizations managing their video monitoring services.
Growing a business is vital in an industry where fierce competition makes it difficult to increase service fees to offset rising costs. AI and other analytics software opens new doors of opportunity to central monitoring stations already heavily dependent on human resources. As skilled labor becomes harder to find and more expensive to employ, these companies can now look to technology to fill gaps.
Other benefits include:
- Higher employee satisfaction and retention.
- Lower recruitment and training costs.
- More competitive customer service level agreements (SLAs).
- Faster deployment time for integrating analytics using remote, cloud-based software.
The Future
What’s next for machine learning as it applies to false alarms? Look for software able to identify relationships between behaviors and criminal intent. This could advance crime prevention by having the software learn what actions most likely lead to illegal activity.
The latest technology makes it easier for remote video monitoring operators to keep sites safe and secure; in fact, during the COVID-19 pandemic, owners of construction sites, warehouses and other facilities have turned to remotely monitored video as a cost-effective means of securing locations when most or all employees are forced to stay home.
While it has taken nearly 10 years of development for software to become capable of significantly reducing false alarms, as platforms continuously learn, expect new advances to come more quickly.
Brian Baker is VP Americas for Calipsa, a provider of video analytics for false alarm reduction. Request more info about the company at www.securityinfowatch.com/21152437.