1.28.22 – SIW
Solution-providers have created more cost-efficient and user-friendly applications for AI video and other platforms
Like the classic Beatles song says, it has been a long and winding road getting to where the security industry is today with advanced video analytics. The video analytics highway has been littered with overblown promises and under-delivered performance this decade. The technology has evolved from motion-based analytics that could handle rudimentary object detection, object recognition and object tracking to solution providers that have offered variations of background subtraction-based analytics, which is usually the first step of video analytics used to extract the interesting focal point of the monitored area.
The most advanced video analytics now incorporates an entirely new approach, leaving behind the challenges of the past and starting anew with the intelligent learning capabilities of artificial intelligence (AI). As the industry moves into this phase of video-enabled AI which is delivering better accuracy and performance than previous solutions users can now index what’s going on in their video in real-time, do it with low latency and high accuracy in a new phase that is being called the second wave of video analytics. In addition, there are innovative opportunities to now run additional AI on all the metadata that AI is producing about what is going on in video. This can find new patterns and events that were previously undetectable to human operators.
Video Analytics Explained
Video analytics may have several different objectives depending on its application, but the overriding goal is to automatically recognize temporal and spatial events in videos and make them actionable to the user. Simple examples may include tracking the action of a person who moves suspiciously in a facility or parking lot, detecting the sudden appearance of flames and smoke, ensuring the safety and security of patients and staff in a healthcare facility, the applications are endless. The benefits are further enhanced by virtue of real-time video analytics and video data mining.
For technologists like Brent Boekestein, who is the co-founder and CEO of Vintra, Inc., a company delivering AI-powered video analytics solutions that helps transform any real-world video into actionable, tailored and trusted intelligence, being able to assimilate and then communicate the patterns, deltas, correlations, and anomalies buried in the vast lakes of video data into actionable risk mitigation responses is what excites him about the exploding potential of deep learning and the vastly improved analytics that its AI features.
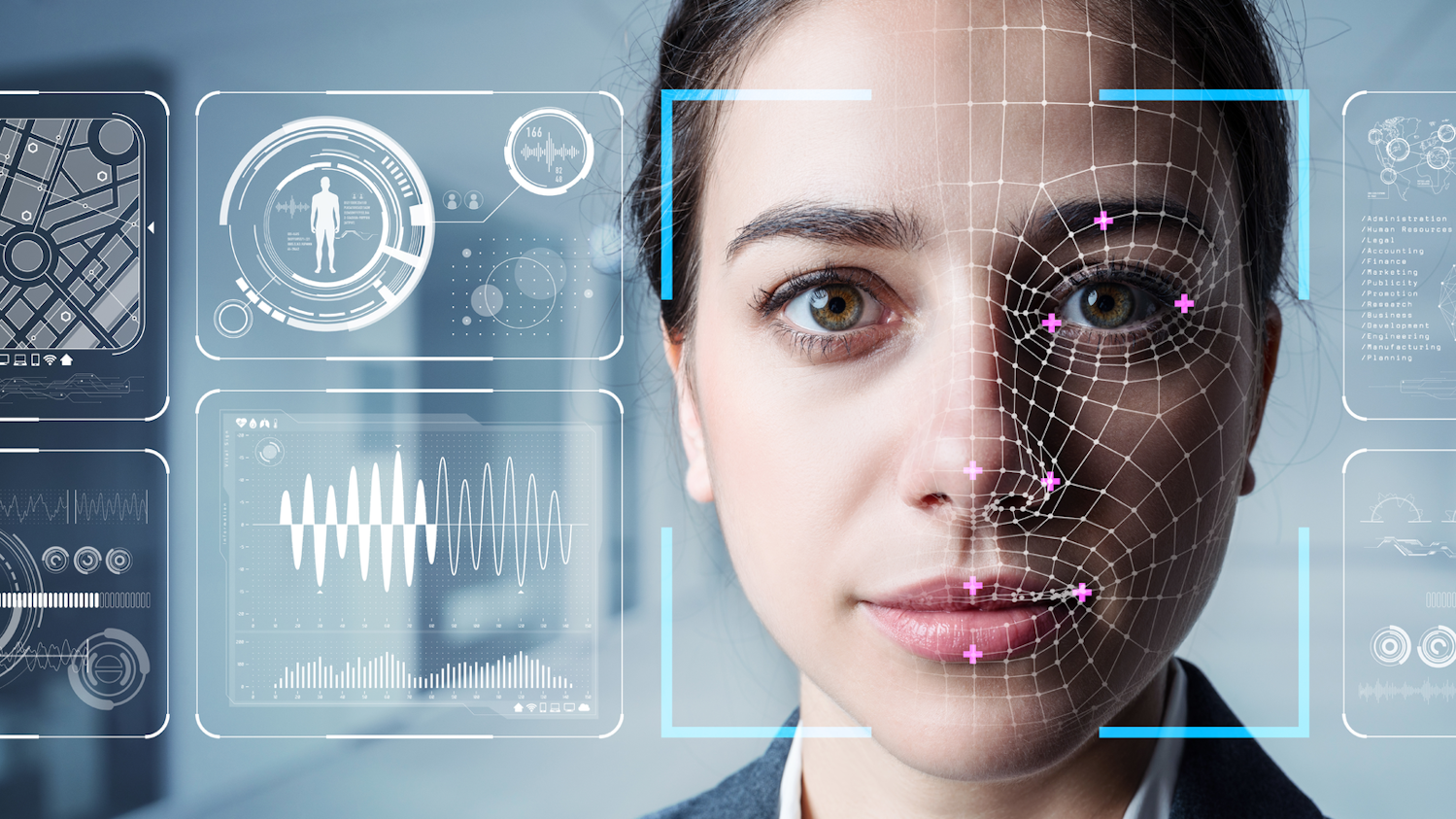
“Today, algorithms can get smarter over time and we {would} always hit a ceiling when you had background subtraction in these older algorithms. You had to fine-tune them by hand. You could dominate the market by being the company that had hand-tuned the healthcare hospital hallway algorithm for slip and fall, but that’s all you could do with that algorithm. You just couldn’t move it somewhere else. You couldn’t add new data to it. It was only for that thing,” explains Boekestein, who adds that the power of deep learning exponentially widens the scope of intelligent video’s impact on overall enterprise-level applications.
He says that his team was pioneering this approach to analytics from the company’s early days and emphasizes Vintra’s leadership role in the use of synthetic training data.
“We’re creating the data to train our algorithms in video games and in virtual environments and seeing a lot of advantages. It’s faster and you can strip the bias out of it because you control what goes into it. It’s immediately labeled perfectly. There are no errors on that front. Plus, we’re seeing that it has an impact on precision and recall rates,” Boekestein continues. “So, why is deep learning taking over? You have better hardware, better training data, better algorithms, and this stuff just continue {to grow}. The acceptance curve for this technology is wonderfully strong right now.”
The Science is the Differentiator
The technologies that were involved originally in analytics for video were challenging because video had to be read frame by frame in the frame processing method, and each frame, each image processing had to be performed to remove features from that frame. You also had to manage several image processing libraries to help manipulate the mixture of three key tasks to perform. The process of justifying object detection, object recognition and object tracking would eventually lead you into real-time video analytics that could trigger real-time alerts.
Some solution providers were simply “slapping AI” into their software applications, says Boekestein, and many are still using open-source detectors, which forces detectors to be retrained in the case of needing to add new categories. As an example, YOLO, the go-to algorithm used by the bulk of security industry analytic providers, doesn’t even come with a face detector in its native open-source version. The algorithm uses neural networks to provide real-time object detection and gained popularity because of its speed, accuracy, and availability. But it also is known to be computationally intensive, difficult to retrain when new classes are needed, and, since it wasn’t purpose-built for the security and safety use cases, has to be augmented with lots of new training data to improve its performance and flexibility.
So according to Boekestein, the AI functionality of these open-source options that many companies use becomes muddled as increasingly open-sourced software options are stacked on top of each other and weren’t purpose-built to work together. For example, ArcFace, whichis a machine learning model used for facial recognition that takes two face images as input and outputs the distance between them to see how likely they are to be the same person, is often combined with YOLO to create a “Frankenstein” solution of an object detector and face rec solution. This piecemeal approach can render the tech behind other company’s video analytics as inflexible, overweight, lower-performing solutions that are not purpose-built. These scenarios can require expensive camera upgrades and can present challenges for the ensuing migration to mobile surveillance. Vintra has taken a different approach by purpose building their own neural networks that are faster, more accurate, and more flexible than open-sourced options like YOLO.
Making the Value Proposition for the Enterprise Beyond Security
“You traditionally had security –, maybe you called it Environmental Health Safety and Security in one place – and your safety brethren were sitting over here but weren’t allowed to look at what you were {monitoring}. Instead, you could get a report every now and then. And that data stayed siloed in that security system,” Boekestein laments, adding that it is an entirely different approach now that allows the sharing of critical operational information to enhance each business sector’s efficiency and security.
“Deep learning has had an interesting effect here. First of all, we can find more things and find them faster and more accurately. All of a sudden, the data becomes more useful, more trusted by those other stakeholders that are not in security. That’s step one. Secondly, AI allows us to quickly customize detectors and make the system even smarter over time. “Step two is what we and others are doing. We ship our tech with a standard set of detectors for faces, whole persons, vehicles, bags, weapons, people falling down and more,” continues Boekestein, pointing out that his solution is also capable of providing easy customized upgrades and alterations for those detectors. “We built it from the ground up, so we can slap in new detectors to that, and it does not impact the compute efficiency, it does not impact the performance. You get to control those things when you built it from the ground up.”
As for the third step in this process, Boekestein continues: “All of a sudden, you’re a security person and your opportunity is to have a return-on-investment conversation and not a cost conversation with the finance team. Solutions like this support our motto that data wants to be free. We have an open API format, and you can’t ask the facilities person to come to your security application and stare at a dashboard with another login, another place to click. You have to bring the data to where they’re making the decision about how to size, service, or ultimately build out that facility if they’re looking for utilization data.”
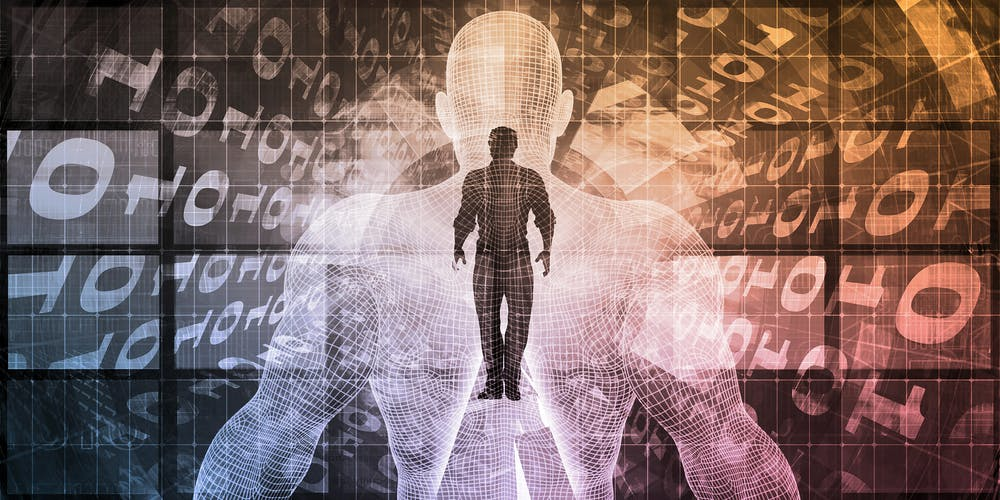
“If the operations team is trying to figure out how many sticking forklifts have to come through this space, we have to know how wide to make the forklift lane at the next facility; you can’t ask them to come to a security application to do that,” says Boekestein. “Data should be like vapor, meaning it should be everywhere that you need it to be, not in a lake that you come to ask these people to drink at, and they’ve got to come to you. That’s how security is going to make itself an ROI center and not a cost center.”
The Vintra Difference
Vintra works on live or recorded video, whether that is from fixed or mobile surveillance cameras. It can be deployed as a standalone technology connected to any IP camera with an RTSP feed or it can be deployed as a deep integration to some of the leading security products in the market. By automatically indexing video streams so they can be alerted on or instantly searched, Vintra adds a “brain” to all the “eyes” (cameras) installed around a facility and delivers operators’ force-multiplying capabilities.
The company’s enterprise-grade solution, Vintra Prevent, supports real-time video typically used by organizations that have GSOCs and their own set of hundreds or thousands of cameras. The solution automatically indexes video streams and enables new security workflows to be created via accurate event alerting and instant search. The Vintra Investigate option, like Prevent, can be deployed in the cloud or on-premise. It is reserved for dedicated investigation teams that undertake a mission-critical, laborious review of the already-recorded, third-party video, which can help mitigate security and safety risks from escalating or even occurring.
As Boekestein puts it, “We focus on both sides of the bang: we provide better situational awareness to better prevent issues, we help teams react more swiftly to real-time events and we dramatically speed up investigative results.”
Building on its purpose-built value proposition and API differentiator, Boekestein believes that his team’s ability to process mobile video is another selling point that enterprise clients have embraced. He says that the growth of camera counts and camera penetration on fixed surveillance is plateauing and is experiencing a couple of percentage points growth a year while mobile surveillance is rapidly growing and reshaping the posture of video surveillance in large organizations.
“Look at what’s happening with drones, dash cameras, body cameras, the evolution of the mobile phone as part of the security posture. We’re not in a ‘see something, say something’ culture anymore. We’re in a ‘see something, send something culture.’ So, all of your stakeholders with a mobile phone can now be part of your security posture,” he contends. “Almost every one of our customers is contemplating or has already deployed {a mobile video solution}. It’s certainly limited today, but we’re selling them a future-proof solution.”
How is AI Impacting Physical Security Today?
From video surveillance to access control, the proliferation of AI-enabled devices across the physical security spectrum has created a paradigm shift in the way end-users collect and disseminate information across various business silos in their organizations. According to Boekestein, one of the most conventional and cost-efficient methods is to convert all of your existing IP cameras to AI-enabled cameras.
“This wasn’t the case a few years ago but the improvement in deep-learning algorithms and hardware has made it so today. And it’s not just fixed cameras, but deep learning unlocks the ability to run analytics on mobile surveillance feeds as well from dash, body, drone, and mobile phone-based cameras. This leads to a few benefits. Investigation time is reduced by 90% since everything that happens on camera is searchable. Also, critical event response time is reduced. We have a banking customer that had an HQ break-in (in the dark with intermittent lighting) and we helped cut their detection and awareness time from a few minutes to a few seconds,” says Boekestein.
Finally, he explains that AI-powered video analytics allow security practitioners to scale their preventative capabilities without scaling their headcount.
“It’s possible to turn every existing camera into a low-latency, high-accuracy, security guard that never quits, goes on vacation, or misses a beat. Since you can detect more (and even customize what you detect) and it’s all stored and available (or should be depending on the vendor) via open APIs, security can become a more powerful business partner to safety, real estate, facilities, and operations teams. They can all leverage the data to make better business decisions about how physical spaces are secured, sized, utilized, and serviced,” he concludes, emphasizing that the benefits of AI-powered analytics are strong as a standalone security solution, but when partnered with business intelligence, it supplies even more insight and organizational advantages.
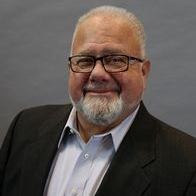
About the Author: Steve Lasky is a 34-year veteran of the security industry and an award-winning journalist. He is the editorial director of the Endeavor Business Media Security Group, which includes magazines Security Technology Executive, Security Business and Locksmith Ledger International and top-rated webportal SecurityInfoWatch.com. Steve can be reached at slasky@endeavorb2b.com.